Research: artificial intelligence to help learning in conflict zones
Feb. 23, 2021
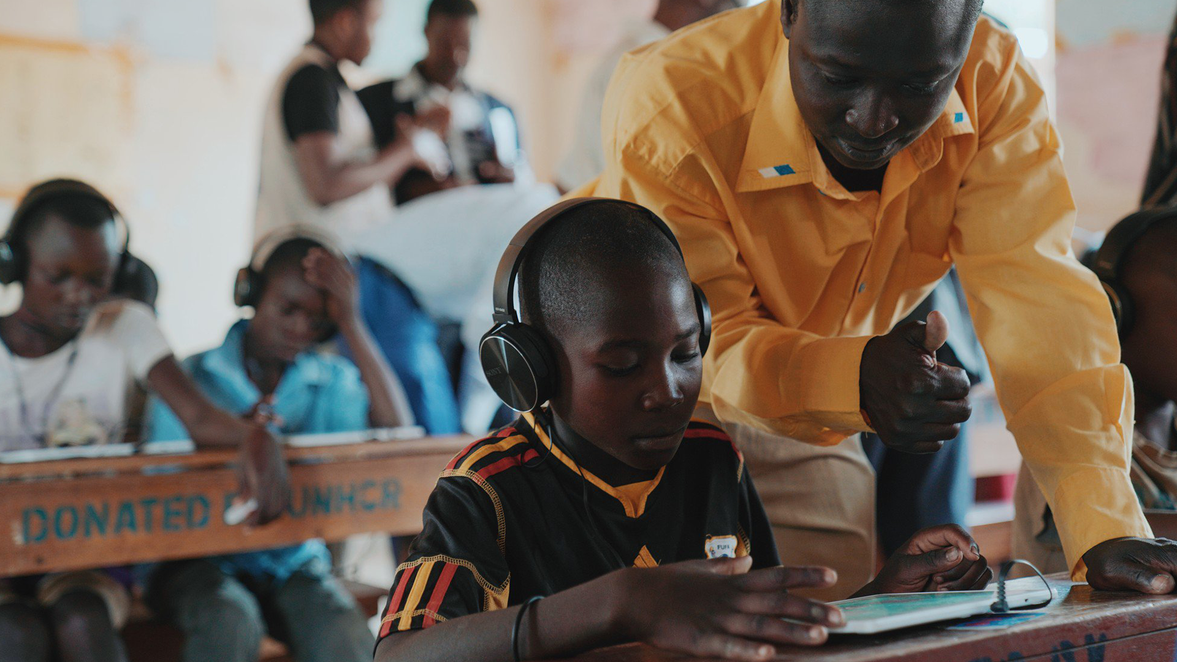
Some 75 million children affected by armed conflict worldwide currently experience significant disruption to their education. Can’t Wait to Learn - our award-winning Ed Tech innovation - enables children like these to access quality education through digital tablets in difficult settings where professional teachers are in short supply.
Wheel-spinning
Can’t Wait to Learn sees children learn through engaging with a series of mini-games. Maintaining motivation is core to the methodology. There is a pre-defined linear pathway of progress, but sometimes children get stuck on a certain activity. Repeated failures to master a skill in a timely manner is defined as ‘wheel-spinning’. There are several reasons a child may not be able to complete a mini-game. For instance, if too much time passed since the last time they played, they may forget the required skills to complete the mini-game.
Learning barrier
Wheel-spinning is a problem because it can be demotivating for children, and hamper learning progress. Researchers from Stanford University and War Child Holland examined this aspect of the programme.
We found that only 2.4% of student problem pairs demonstrate wheel-spinning, 51% of children experience wheel-spinning at least once. Student classes are often very large: when wheel spinning occurs, the child may have to wait until a teacher or learning facilitator finds the opportunity help them.
What did we learn?
The research findings suggest that Machine Learning - a branch of artificial intelligence based on the idea that systems can learn from data and make decisions with minimal human intervention - can identify the reason a child is stuck on the Can't Wait to Learn mini-games and would be able to select an actionable intervention. We compared the machine learning's evaluation with the opinion of an expert, and machine learning was on par with the expert. For example, machine learning could predict if the issue was with the instruction, content, pre-requisite skills or an issue with the amount of time that had passed between last playing the game. In the future, if this intelligence can be added to the game, it can also identify the activity the learners must do to prevent getting stuck. For instance, the game could instruct the child to jump back and revise the necessary skills needed to progress in the mini-games.
Improved learning
This way the tablet could better facilitate learning and intervene automatically, instead of waiting for a teacher or facilitator to help. At scale this could save students hours and hours of time and keep motivation high. Ultimately, Machine Learning has the potential to bring about improved learning and numeracy learning outcomes within Can’t Wait to Learn.
Publication: Tong, M., Jetten, A., Brunskill, E. (2020) Towards Suggesting Actionable Interventions for Wheel-Spinning Students. [Paper presentation] Proceedings of the 13th International Conference on Educational Data Mining: Online